Data runs everything in today’s business world. You know this if you’ve ever tried making decisions without good data. It’s like driving with your eyes closed. I’ve seen companies waste thousands of dollars because of bad data. The costs add up fast. AI now changes how we handle data quality. Gone are the days of manual spreadsheet checks and endless data cleaning. AI tools catch errors humans miss. They work around the clock without coffee breaks. Quality data leads to better decisions. Poor data creates expensive mistakes. AI helps bridge this gap with speed and accuracy that humans can’t match. The transformation happens right now across industries. Here are tips on how AI data quality management redefines accuracy and efficiency.
Importance of Data Quality Management
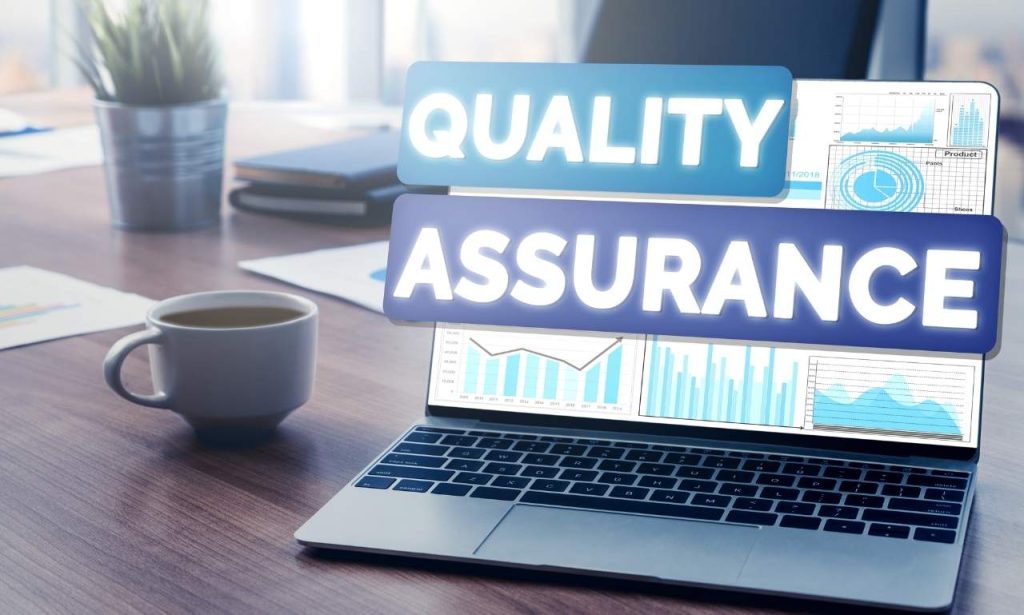
Data quality impacts every part of your business. Bad data equals bad decisions—it’s as simple as that. I’ve watched companies tank campaigns because of outdated contact lists.
Customer trust depends on accurate information. Imagine sending birthday offers to deceased customers. One retail client lost $50,000 on a campaign targeting incorrect segments, and the marketing director nearly lost his job.
Regulatory compliance requires clean data. Financial penalties for data mistakes grow each year, so your team needs systems that prevent costly errors before they happen.
Data quality directly affects your bottom line. Harvard Business Review reports bad data costs U.S. businesses $3 trillion yearly. Most executives don’t realize how much they’re losing. The numbers would keep them up at night.
Good data quality management creates competitive advantages. It reveals opportunities competitors miss. My most successful clients prioritize data quality as a strategic asset.
Traditional Data Quality Management vs. AI-powered Solutions
Traditional methods rely heavily on manual processes. Teams spend countless hours checking spreadsheets for errors. I’ve witnessed data analysts waste entire weeks on tasks AI completes in minutes.
Rule-based systems catch only known issues. They miss complex patterns hiding in plain sight. One manufacturing client discovered thousands of billing errors, and their systems were never flagged.
Human error plagues traditional quality management. Even careful workers make mistakes when processing large datasets, and fatigue and boredom guarantee oversight problems.
Scaling traditional approaches costs too much. Adding more data analysts doesn’t solve the fundamental problems, and the ROI simply isn’t there anymore.
AI solutions transform this landscape completely. Machine learning identifies patterns invisible to human workers, and neural networks adapt to new data quality challenges without reprogramming.
AI tools process millions of records in seconds. They work tirelessly without complaints or vacation days, and the efficiency gains create immediate ROI for most companies.
Automated anomaly detection catches problems before they spread. AI systems learn from each correction, growing smarter over time. This continuous improvement cycle doesn’t exist in traditional systems.
Real-time monitoring prevents bad data from entering systems. Problems are fixed at the source rather than downstream, saving countless hours and dollars.
Key AI Technologies Driving Data Quality Transformation
Let’s explore the technologies that are reshaping data quality management. Machine learning algorithms detect patterns that humans would never find. They identify relationships across thousands of variables simultaneously.
Machine learning models improve with more data. They become increasingly accurate without manual intervention. This self-improving cycle creates exponential value over time.
Natural Language Processing transforms unstructured text into usable insights. It extracts meaning from comments, reviews, and documents automatically. The technology turns messy text data into structured gold.
NLP handles multilingual data seamlessly. It normalizes information across languages and formats. This capability proves invaluable for global companies managing diverse data sources.
Computer vision technology validates visual data quality. It identifies problems in images, videos, and visual records. This matters increasingly as visual data grows in importance.
Deep learning networks handle complex quality issues. They find subtle patterns indicating data corruption or inaccuracy. Technology excels at solving problems that are too nuanced for simpler approaches.
Automated data cleansing tools fix problems instead of just flagging them. They standardize formats, remove duplicates, and correct obvious errors. The best systems explain their changes for human review.
Cloud-based quality platforms scale instantly with demand. They handle seasonal spikes without expensive hardware upgrades. This flexibility delivers consistent performance regardless of data volume.
Benefits of AI-driven Data Quality Management
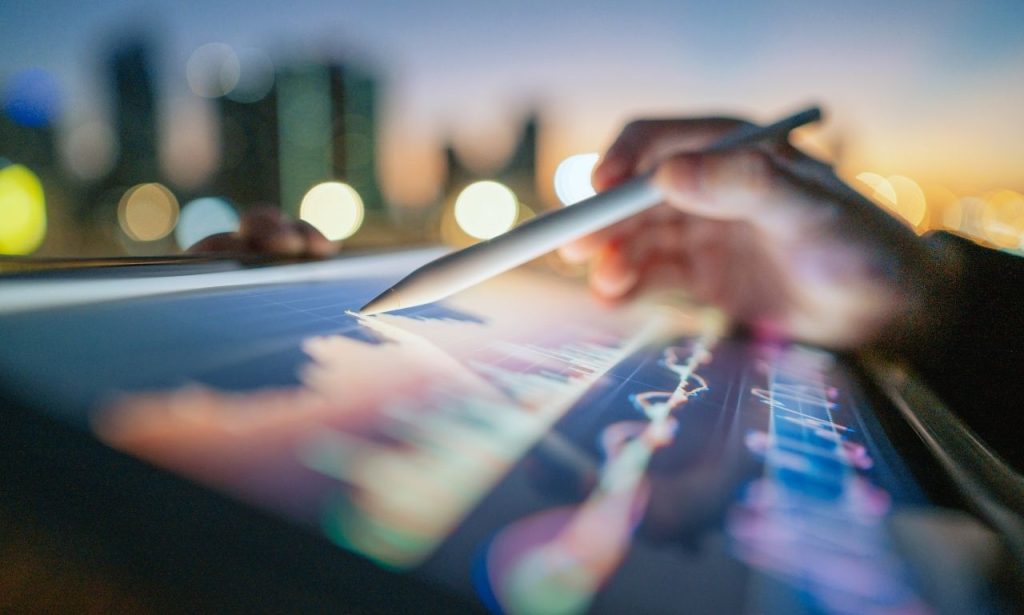
AI quality management delivers measurable time savings. Tasks that took weeks are now finished in hours or minutes. A financial services client reduced reporting time from five days to six hours.
Cost reductions appear immediately after implementation. Most companies see 30-60% lower data management costs. These savings come from both labor and error prevention.
Accuracy improvements transform decision quality. With clean data, I’ve seen prediction accuracy jump from 70% to 94%. Better inputs create dramatically better outputs.
Real-time monitoring prevents costly mistakes. Problems get caught before they affect downstream systems. One retail client avoided $200,000 in inventory errors through early detection.
Regulatory compliance becomes less stressful. AI systems document data lineage and quality measures automatically. This audit trail proves invaluable during regulatory reviews.
Accurate data improves customer experiences. Marketing becomes more relevant, and service becomes more personalized. Customers notice and reward companies that get the details right.
Competitive advantages emerge from superior insights. Companies make better decisions faster than rivals, and this speed and accuracy translate directly to market share gains.
Trustworthy data improves resource allocation. Teams invest where opportunities exist, not where bad data suggests. This optimization often delivers the largest ROI.
Challenges in Implementing AI for Data Quality
Implementation challenges exist despite clear benefits. Legacy systems often resist integration with modern AI tools, and workarounds cost time and money during transition periods.
Data silos create blind spots for AI systems. Information trapped in departmental databases limits effectiveness. Breaking down these walls requires both technical and organizational changes.
Skill gaps slow adoption in many companies. Teams need training to work effectively with AI tools. This learning curve can temporarily reduce productivity before improvements kick in.
Cost concerns deter some organizations despite clear ROI. Initial investments seem high compared to maintaining the status quo. Short-term thinking creates long-term disadvantages.
Trust issues affect user adoption rates. Some teams resist trusting AI recommendations. Building confidence requires transparency and early wins to demonstrate value.
Cultural resistance sometimes emerges from data teams. People fear job displacement despite evidence showing changing responsibilities, not elimination. Change management matters as much as technology.
Privacy regulations create implementation hurdles. Systems must balance effectiveness with compliance requirements. This balancing act adds complexity to quality management efforts.
Measuring success requires new metrics. Traditional KPIs don’t capture the full impact of AI-driven quality. Companies need updated measurement frameworks to demonstrate true value.
How AI Is Transforming Data Quality Governance Across Industries
Finance
Financial institutions handle millions of transactions daily. AI quality tools detect fraud patterns in real time. They flag suspicious activities that rule-based systems miss entirely.
In banking, AI validates customer information automatically. It checks addresses, phone numbers, and identity documents. This automation reduces onboarding friction while improving compliance.
Investment firms leverage quality management for better predictions. Clean data leads to more accurate market forecasts. Even minor accuracy improvements create enormous value in this sector.
Insurance companies use AI to verify claims information. The technology validates documents, images, and submitted details. With AI quality checks, fraud detection rates improve dramatically.
Credit decisions rely increasingly on AI-verified data. With trustworthy information, lenders make better risk assessments, and default rates drop when decisions use properly validated data.
With AI oversight, regulatory reporting becomes more reliable. Financial institutions avoid costly compliance penalties, and the technology creates audit trails that prove due diligence in data handling.
Healthcare
In healthcare, AI verifies patient information accuracy. It prevents dangerous medical errors from insufficient data. Patient outcomes improve directly through better information quality.
AI quality management benefits electronic health records. The technology standardizes formats and identifies contradictions, creating more complete patient profiles for better care.
Insurance claims processing improves through automated validation. AI systems check procedural codes and treatment documentation. They catch billing errors before submission.
Clinical research relies on clean, validated data. AI tools ensure that study information meets rigorous standards, improving research quality and leading to better medical advances.
Pharmacy systems use AI to verify medication information. The technology prevents dangerous prescription errors. It checks dosages, interactions, and patient-specific risks.
Healthcare providers predict resource needs more accurately. Clean data leads to better staffing and supply management. This optimization reduces costs while improving care quality.
E-commerce
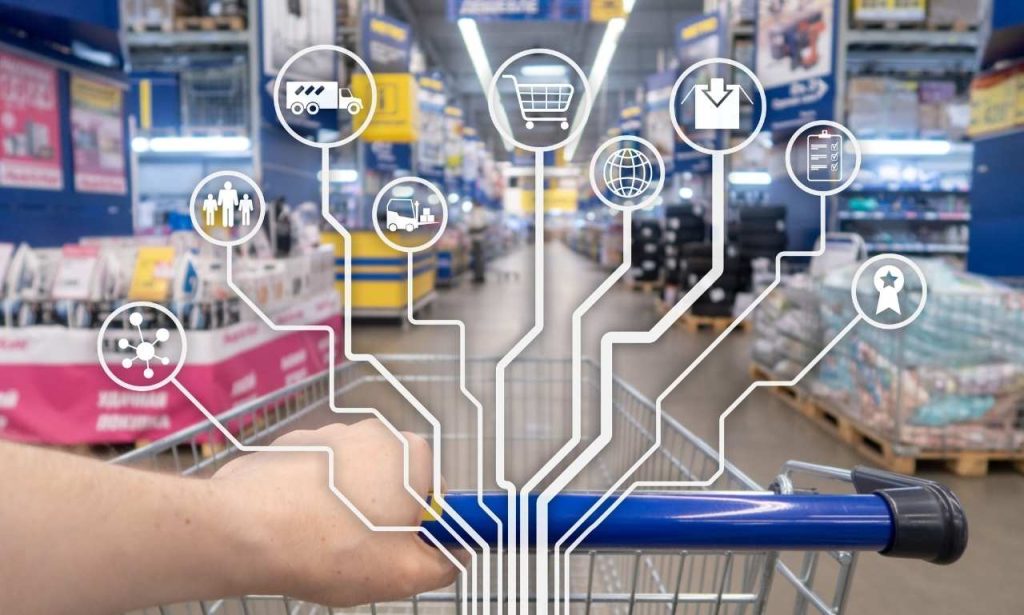
E-commerce companies transform customer experiences through data quality. AI validates product information across thousands of listings, ensuring customers find exactly what they’re seeking without frustration.
Inventory management improves dramatically with AI oversight. Systems predict stock needs based on trustworthy sales data. Stockouts and overstocking both decrease, improving profits.
Personalization engines work better with clean data. Recommendations become more relevant and compelling. Conversion rates climb when customers see truly appropriate suggestions.
Pricing optimization relies on accurate competitive information. AI tools verify competitor data before analysis. This validation prevents costly pricing mistakes based on bad inputs.
Customer service improves through AI-validated information. Support teams access accurate purchase and return histories. Resolution times decrease while satisfaction scores climb.
Quality management tools benefit logistics operations. Verified address information improves delivery routing, decreasing late deliveries and creating happier customers.
Conclusion
AI data quality management transforms how businesses handle information. The technology delivers accuracy and efficiency, but human-only systems can’t match. Companies gain competitive advantages through better decisions.
Implementation challenges exist but pale in comparison to the benefits. Forward-thinking organizations already leverage these tools successfully, gaining market share while others hesitate.
The future belongs to companies that prioritize data quality. AI makes this possible at scales previously unimaginable. Your organization faces a choice: lead this transformation or follow competitors.
I’ve witnessed dramatic improvements across numerous clients. Those embracing AI quality management consistently outperform peers. The technology proves its value through measurable business results.
The time for waiting has passed. AI data quality management isn’t theoretical—it’s practical, profitable, and available today. Your data deserves nothing less than the best tools available.
Also Read: How Address Verification Helps Prevent Fraud
FAQs
AI systems process more data faster, learn from corrections, and identify complex patterns that humans miss.
Most organizations see initial results within 2-3 months, with full implementation taking 6-12 months, depending on complexity.
Finance, healthcare, and e-commerce dramatically improve, but all data-driven businesses benefit.
Most companies report 30-60% cost reductions through automation and error prevention.
Basic data literacy and tool-specific training, with data scientists needed for customization but not daily operation.